Tackling Climate and Water Challenges in the Himalayas using AI
- Suraj Dalal
- Sep 17, 2020
- 5 min read
Updated: Sep 20, 2020
Written and photographed by Suraj Dalal
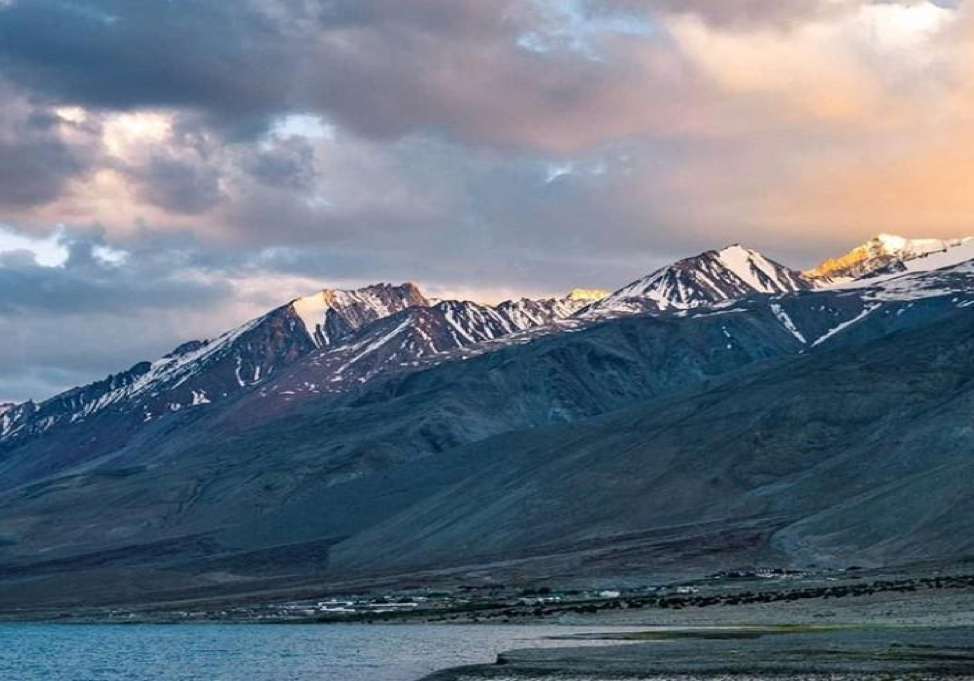
Pangong Lake, Ladakh
The unprecedented and existential challenges of climate change are gaining attention. However, the scale of innovation required to manage the crisis doesn’t receive as much recognition. Against this background, new technologies such as Artificial Intelligence (AI) hold promise in helping tackle the climate and water challenges most pertinent to the vulnerable populations in the Himalayas. This article explores one fundamental question: how can we deploy existing and emerging technologies to undertake ambitious climate action in the Himalayas?
Leveraging Data in the Himalayas
The term ‘machine learning’ is often used as a panacea to solve the pressing challenges of the world. This narrative is far from the reality. Machine learning requires human designed algorithms. Studying data involves identifying patterns from existing information and using it to predict meaningful outputs, be it in the form of solutions or problem diagnoses. The field of environmental and climate science utilizes enormous amounts of cryosphere data. Access to credible data on the Himalayas is limited, and existing data needs to be complemented with rapidly evolving algorithms to produce meaningful results.
Large geoscience datasets which include seismic data surveys, and supercomputer simulation outputs from global climate model projects are now available in the public domain. Using AI, open source tools and cloud computing, we can convert a large amount of data about nature into valuable information that can inform policy choices.
Machine learning is utilized to generate insights from satellite imagery and to detect and predict changing geographic patterns. One way this technology is used is by taking satellite images in the winter, mapping them onto 3D models and comparing between two seasons. The findings help in predicting the volume of snow that will melt in the summer and help us measure the size of ice sheets, glaciers, and snow cover. Over a period of time, this data can be used to show historical snow dips and predict snow cover, delivering much more accurate information that can inform preparedness and response to potential climate-related events.
One such use is real time monitoring of snow and ice flow. In 2019, Researchers at Columbia University and the University of Utah used newly accessible images taken by US spy satellites between 1975 and 2000 that captured a 2000 km-wide stretch of the mountains across India, China, Nepal and Bhutan. They converted them into 3D models and compared them against present-day satellite images of the same areas. The findings were startling. From 2000 to 2016, glaciers lost equivalent to more than vertical foot and half of ice each year due to rising temperatures.
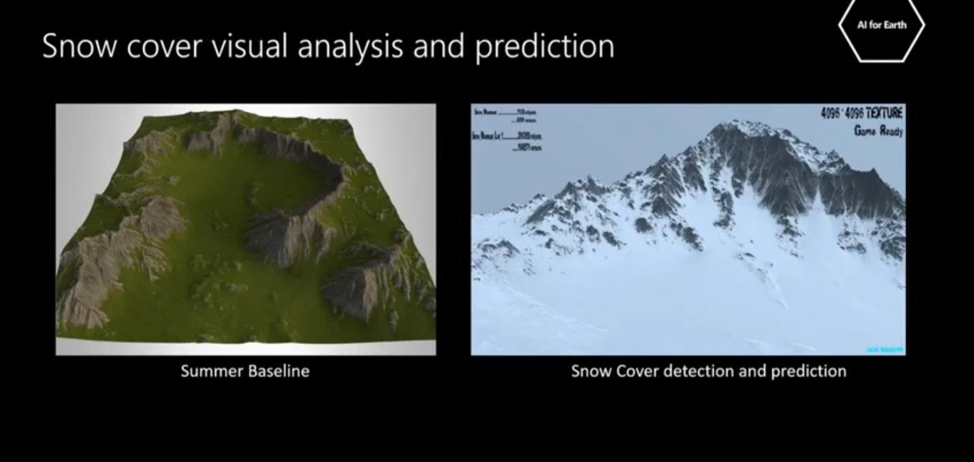
Figure 1. Comparative analysis of summer and winter snow cover
Existing Initiatives and Solutions
The global research community has made unprecedented breakthroughs in utilizing data and machine learning to understand melting ice-caps across all polar regions. A team of scientists from the Montreal Institute for Learning Algorithms (MILA) used Generative Adversarial Networks to generate images predicting how climate events may impact our environments. They demonstrate techniques ranging from unsupervised learning to increase the accuracy of ice flow monitoring in multi spectral satellite images, and tracking ice sheet and glacier dynamics.
The prediction of future ice melt and tracking the optical flow of ice dynamics presents significant challenges. This is due to uncertainties in various parameters such as global temperature increase, changing precipitation patterns, occlusion from cloud cover, and rapidly melting and retreating glaciers. This requires continued access to data for better understanding as well as confidence in the efficacy of the existing models. In the figure below, the first three columns are the subscenes provided to the algorithm and machine learning predicts the next subscene. There is a consensus to encourage the AI community to contribute to the study of climate change and develop new models on different types of terrains.
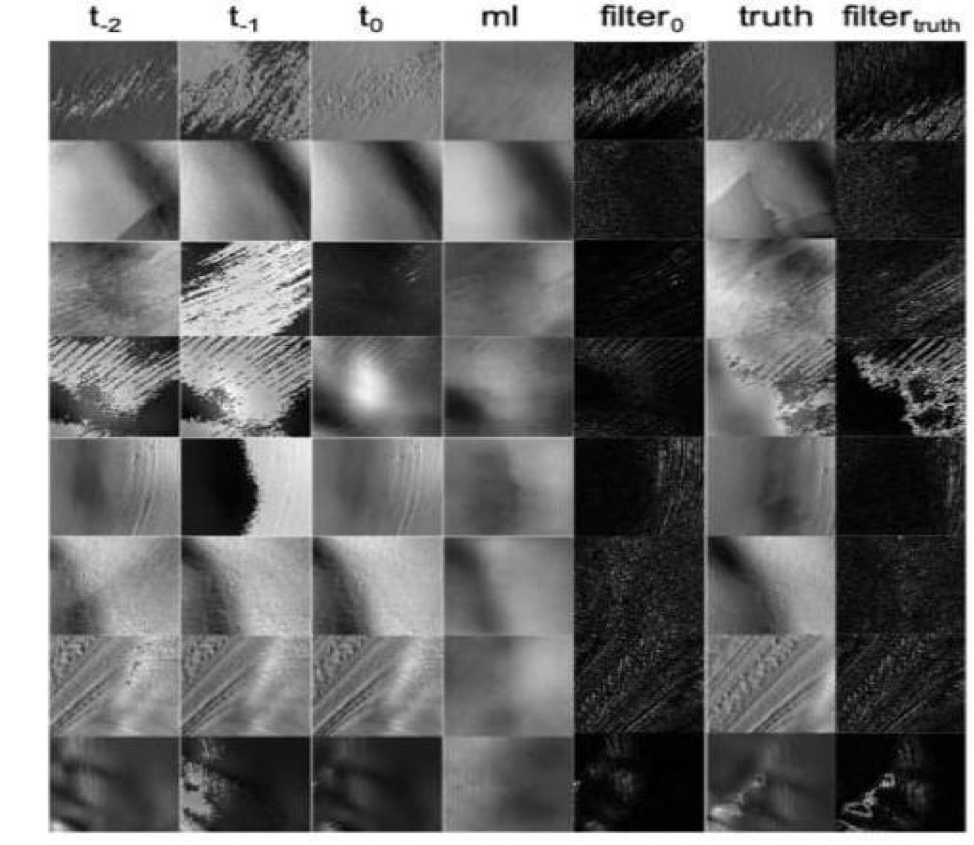
Figure 2. t2, t1, t0 are the past three subscenes, fourth column shows next subscene predicted by machine learning, fifth column is high pass of t0, sixth and seventh column: the subscene on t1 and t2
The International Center for Integrated Mountain Development (ICIMOD) is the leading research institution in the Himalayan region. Over the years, the center has served as the regional intergovernmental learning and knowledge sharing center, and has made significant strides in the study of climate science in the Himalayan region. For instance, they leveraged data to provide maps, satellite data, expert recommendations and recently issued real time alerts through an early warning system operating in two areas prone to floods: Assam, India and Koshi, Nepal. Villagers receiving alerts had a seven-hour lead-time before the flood hit. In Dihiri, Assam, communities were able to save assets worth USD 3,300 during the flood on September 5, 2013. On August 3, 2017, at 4:30 am, the warning system generated a siren that woke up 2,800 people in the 350 households of Sherkilla. Within an hour, the community had moved about 2,000 heads of livestock and precious belongings to a safe place before the floods entered the village.
Among other research, ICIMOD applies location intelligence software to produce large-scale maps that integrate data on natural resources, lifestyle, and economic factors. These maps show details such as precise watershed boundaries for each Himalayan village, so locals can avoid building houses in high-risk areas. ICIMOD also works closely with local communities and officials to highlight the benefits of mapping in estimating loss and damage. Over time, the maps and data help chart monsoon flood progressions and patterns.
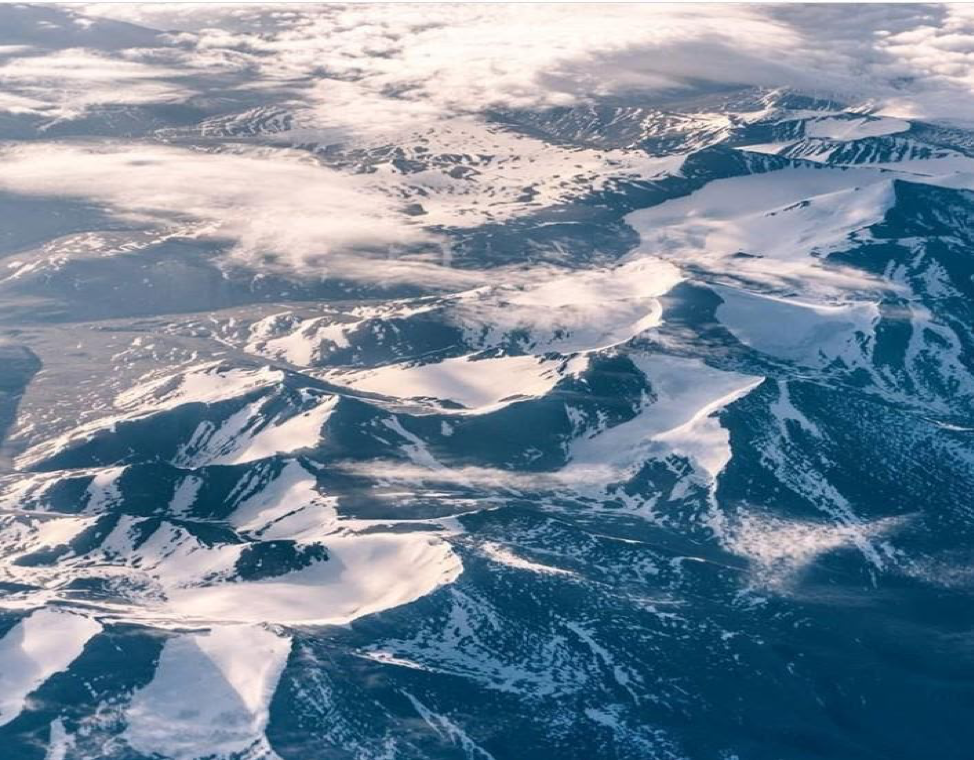
Figure 3. Uttarakhand, India
Landscape Moving Forward
Combining local climate projections with historical observations yields a highly localized picture of future climate impacts. Stories about melting ice packs can be understood much more clearly using satellite imagery.
The onus of climate research in the Himalayas lies not just with nations or research institutes but also with e-tech giants like Baidu, Tencent, Infosys, Alibaba. Tech corporations from countries in the region are increasingly creating their presence in the global community. However, they also need to seek inspiration from Western counterparts like Microsoft, Google and IBM who are actively investing in AI for the cause of climate action and social good. Greenpeace’s recent report shows lack of any advances in clean energy by Alibaba, Tencent and GDS. The tech companies have both financial capability and a staff of really smart people who can contribute to the issue. While companies like Microsoft, Google and Apple setting their targets for carbon neutrality there haven’t been any such pledges by the south Asian tech companies. They should actively invest and fund the sustainability projects and deploy their years of research in the field of artificial intelligence in the area of climate change.
For researchers, there needs to be more effort in leveraging past data into proper algorithms to create meaningful solutions that can aid policymakers. A notable example is Google partnering with India’s Central Water Commission to collect the data needed to roll out flood warnings. This data along with their significant computational power creates better forecasting models to predict when and where floods will occur. Parameters like historical events, river level readings, terrain and elevation of a specific can be fed into algorithms.
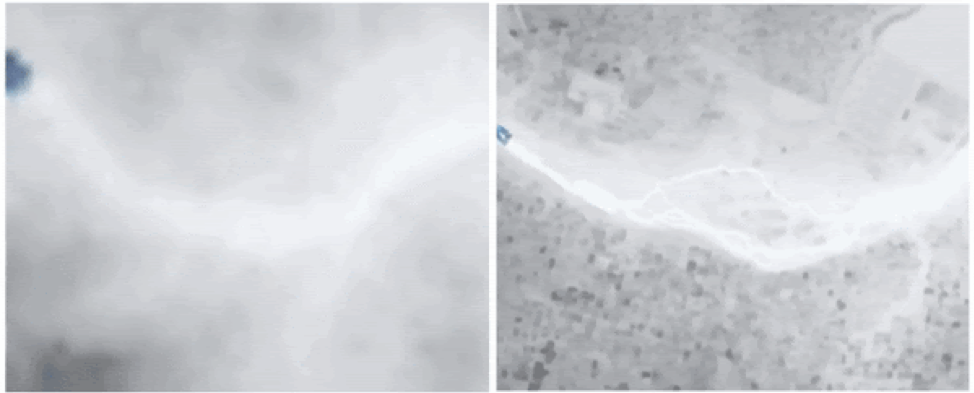
Figure 4. A flood simulation of a river in Hyderabad, India. The left side uses publicly available data while the right side uses Google data and technology. These models contain higher resolution, accuracy, and up-to-date information. Source: ai.google/social-good/
Research on the Himalayas at universities is scant. Governments should promote research by providing researchers with additional infrastructure and funding. Collaboration between government organizations and researchers should take place to facilitate accurate data collection. Notable examples of support to researchers include Microsoft’s “AI for Earth” and Google’s “AI for Social Good” that offer grants to support projects that use AI to solve problems in the field of climate, agriculture, biodiversity and water supply.
Collaboration between various government bodies and tech companies, backed by research institutions with the support of local communities, can strengthen climate action in the region.
Conclusion
Machine learning is not a silver bullet for all climate related challenges in the Himalayas. There needs to be a coordinated effort amongst stakeholders including researchers, universities, tech companies and governments across regional and national levels. Machine learning can empower policy with better data insights to make well-informed and sustainable policy choices.
Suraj Dalal is from India and is an incoming researcher on Machine Learning at Tsinghua University, China.
Commentaires